This first learning package provides a straightforward introduction to the basic elements of AI for a non-tech audience, as well as explains AI’s impact on socio-political and human rights, with a focus on civic space. It is designed to make it easier to explore topic-based or community-specific modules on the ECNL Learning Center.
By the end of this learning package, you will:
- Understand the basics of how AI systems work;
- Have increased knowledge of the capabilities and limitations of AI systems;
- Be familiar with the main overlapping issues between socio-political and human rights aspects in relation to AI;
- Have an understanding on how the definition of AI is used both technically and ‘politically’;
- Be able to debunk common narratives and definitions of AI.
Artificial Intelligence (AI) is a very trendy term. Every tech company wants to be developing AI, while governments all around the world want to invest in innovative technologies. In public debate, AI is often met with two extreme reactions: either with enthusiasm that AI will fix all our problems and make everything more efficient. Or – on the other end of the spectrum - with fear of human erasure by AI robots taking over. Often these attitudes are fuelled by popular representations of AI as humanoid robots, which look like and behave like humans.
But what are we really talking about when we talk about AI? What are the actual benefits and challenges to human life and human rights?
As AI-researcher McKane Andrus explains in the video below: from tech developers, researchers and lawyers, no one is really sure. However, we can distil key elements of various definitions.
Artificial Intelligence is a machine-based system that:
- has the capacity to process data and information in a way that resembles intelligent behaviour;
- analyses, reasons about, perceives and learns about its environment;
- produces outputs, such as predictions, recommendations and decisions;
- for a set of specific (human-defined) goals/objectives;
- with varying degrees of autonomy;
- AI systems may include several methods, such as, but not limited to:
- machine learning, including deep learning and reinforcement learning;
- machine reasoning, including planning, scheduling, knowledge representation and reasoning, search and optimisation.
Often when we talk about AI technologies, we use a couple of terms somewhat interchangeably, especially AI, algorithm and algorithmic decision making (ADM).
The difference between them is that AI is based on algorithms, but not every algorithm is an artificial intelligence system.
ADM on the other hand is usually used to describe systems that make or support decisions about people, for example, predict the risk of fraud or decide if someone should get a loan. They can be based on AI systems or not.
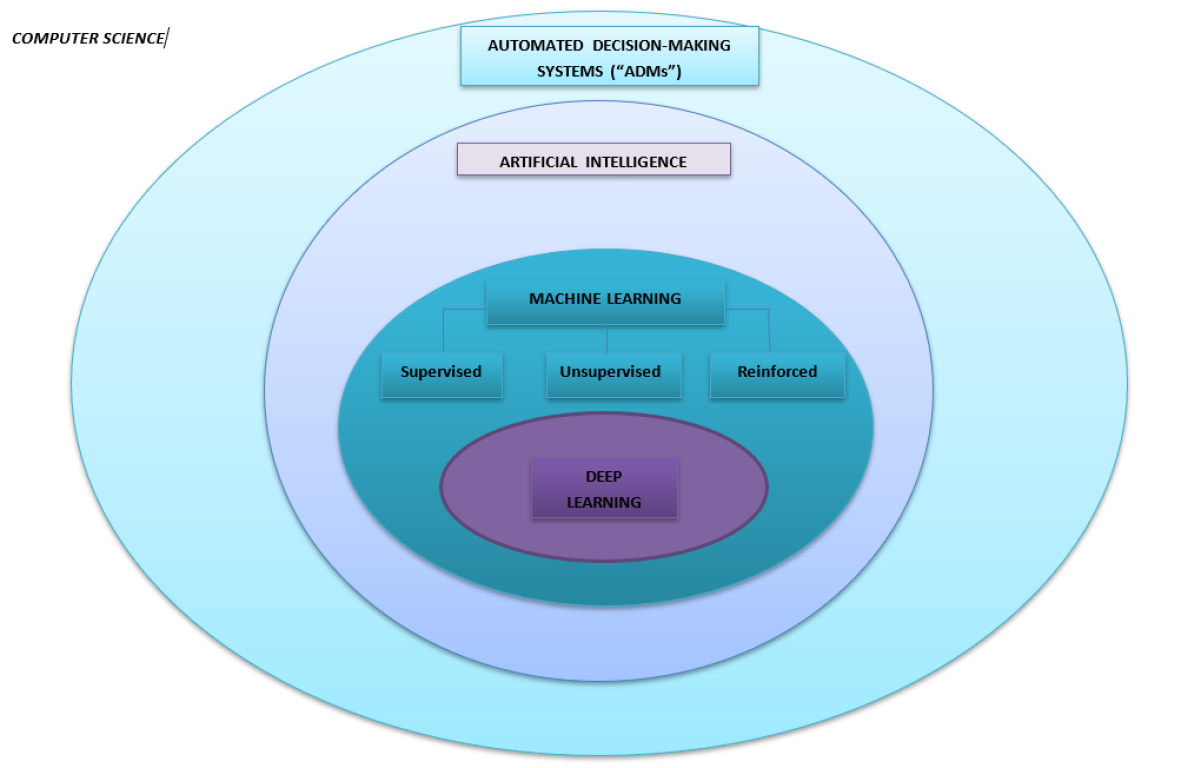
ADM is a wider term than AI. Within AI there is an important and popular technique, called “machine learning”. These terms – AI and machine learning – are used interchangeably in popular discourse. Data scientists refer to machine learning rather than AI because it is considered more accurate in technical terms. So what, then, is machine learning?
Machine learning uses historical data and a definition of success, defined by the team that is developing the system, to find patterns or ways that lead to that success.
There are 4 key stages in the development of a machine-learning system:
- A decisive step is to define the objective of the system and success: what problem are we trying to solve and how will we know that we solved it? This step creates the conditions for everything else;
- Once this is done, designers decide which data they will use for training the system, namely teaching it to recognize patterns;
- Next, under human supervision, or without, the AI system analyses the data to find patterns between them, based on the task it was given;
- And finally, designers evaluate if the system is making good predictions, using a different set of data than that on which the system was trained.
Sounds good, right? What can possibly go wrong? 😊 Well, Andrus explains one example where things went in a very different direction than intended.
The Amazon example:
- To make hiring processes less biased and faster, Amazon deployed a machine learning algorithm to scan CVs and invite candidates for interviews.
- Result: the tool systematically discriminated against women applying for technical jobs.
- The existing pool of Amazon software engineers is overwhelmingly male, and the new software was fed data about those engineers’ resumes. If you simply ask software to discover other resumes that look like the resumes in a “training” data set, reproducing the demographics of the existing workforce is virtually guaranteed.
- For example, the tool disadvantaged candidates who went to certain women’s colleges presumably not attended by many existing Amazon engineers. It similarly downgraded resumes that included the word “women’s” — as in “women’s rugby team.” And it privileged resumes with the kinds of verbs that men tend to use, like “executed” and “captured.”
It is important to question and analyse the data used for training and deploying the system (“input”) and the ultimate goal of the system (“output”).
Based on the explanation above, here are some conclusions that help you debunk certain myths, or rather clarify some general unknowns, about AI.
- “AI has agency”: No AI system, no matter how complex or ‘deep’ its architecture may be, pulls its predictions and outputs out of thin air. All AI systems are designed by humans, are programmed and calibrated to achieve certain results, and the outputs they provide are therefore the result of multiple human decisions. Claiming that AI has agency serves only for diffusing accountability when use of an AI system or algorithm leads to harmful outcome, therefore making it more difficult to hold people accountable and allow for redress.
- “AI is a black box / too complicated”: This argument refers to the ‘lack of possibility’ to trace exactly the system that produces a certain decision. And though this can be the case for complex neural networks (type of machine learning), which we can see as a technical black box, opacity is often a choice that is convenient for different sets of reasons. We see this ‘black box argument’ used to, for example, hide controversial political decisions or protect trade secrets and intellectual property or other corporate interests. However, even when we cannot trace every single technical operation, we can still ‘open up’ human decisions and design choices.
- “AI is objective and neutral”: The Amazon example above shows this is not true. In fact, these statistical and computational biases are mere the tip of an iceberg, resting on deeper layers of biases in our societal context. Such as human biases of, for example, developers’ unconscious prejudices but also systemic biases, in which institutions operating in ways that disadvantage certain social groups.
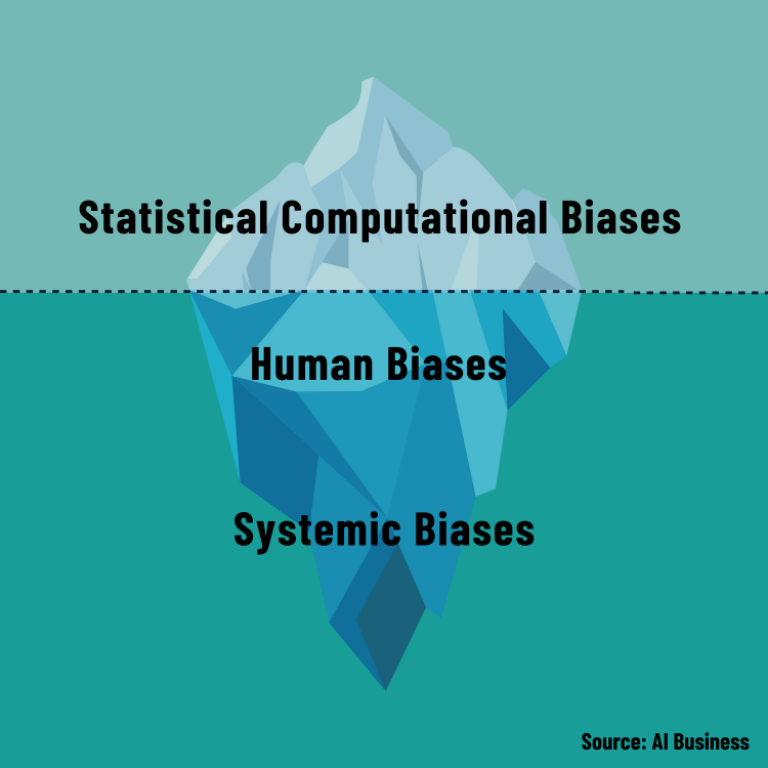
Andrew Strait’s video summarises key challenges of AI specifically for human rights, democracy and the rule of law principle with illustrative examples. The video clearly highlights the connection between human and civic rights.
In summary, the key challenges are:
- Short duration between research and productisation, which makes the barrier for harmful outcomes much lower than other types of technologies that require more investment and more expensive tools to create. It also makes anticipation of harms harder and thereby also regulation and oversight.
- AI systems often make opaque processes even more opaque, which makes contesting those systems more challenging and thereby understanding impacts on human rights and rule of law.
- AI systems operate at extreme scale and speed. More clarity is needed to operationalise existing laws and regulations for AI to protect human and civic rights.
- Systems are dynamic and learn from historic data, which means that even when the AI system is developed and deployed with the best intentions, it can still cause harms when applied.
- Elements of AI by Reaktor and the University of Helsinki is a free online course that teaches the basics of AI and explains its capabilities and limitations. The modules combine theory with practical exercises and can be completed at the participant’s own pace. We especially recommend Part 1 (“Introduction to AI”) and chapters 1 (“What is AI?”) and 4 (“Machine Learning”).
- AI 101 by Aspen Digital gives you an overview without using technical terms and explanations while giving the reader examples on how to write about AI without misleading others.